An Ultimate Guide Into Personalized Recommendations & How To Use Them
Personalized product recommendations are far beyond complementary products and the best-selling products. They are game-changers in user engagement, boosting sales, and transforming how we consume digital content. Personalized product recommendations have revolutionized the online landscape to such an extent that over 35% of Amazon's revenue can be traced back to these tailored suggestions. Netflix helps 80% of watchers discover new shows and movies through its recommendation engine, acquiring 75% of its revenue. Let's unveil the magic behind the scenes and determine how implementing personalized product recommendations can change your business.
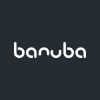
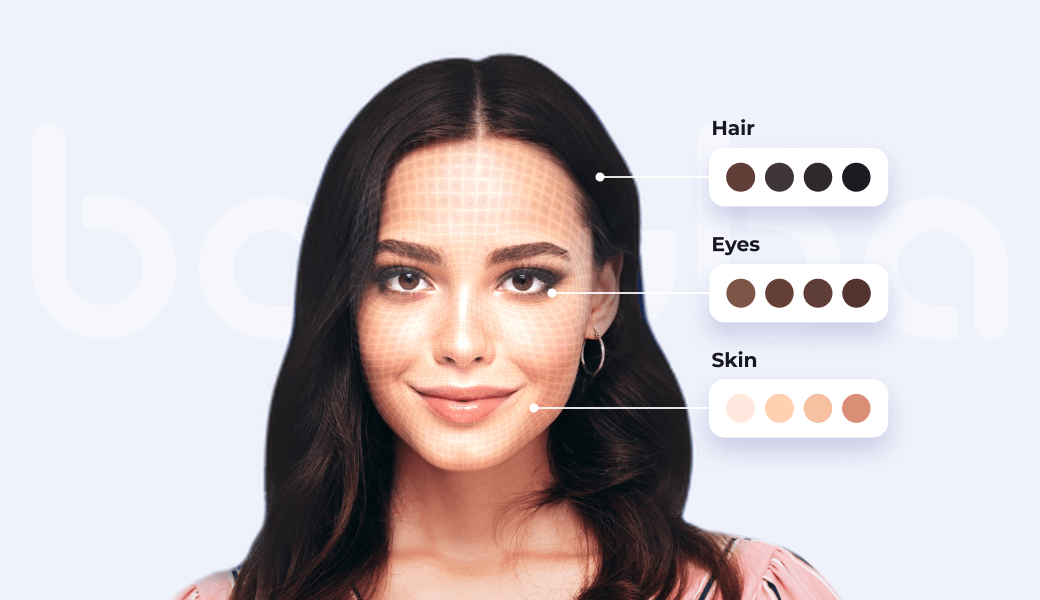
[navigation]
What Are Personalized Product Recommendations?
The online marketplace is as vast as the cosmos; personalized recommendations are the North Star guiding users through the labyrinth of choices. Picture this: every time you shop online, binge-watch your favorite series, or even scroll through your social media feed, the content you see is handpicked just for you. It's like having a personal shopper who knows your desires, quirks, and every click. And 83% of people will be more than happy to share their customer data to receive relevant recommendations in return.
Personalized product recommendations represent a sophisticated mechanism designed to propose items, content, or services tailored to individual users. These recommendations stem from a person's unique preferences, browsing history, prior interactions, purchase history, and user behavior.
At the core, personalized recommendations aim to enrich the user experience and stimulate engagement and conversions. The linchpin is the system's ability to decipher each user's idiosyncratic preferences, leading to tailored content or product suggestions that resonate.
How do Personalized Recommendations Work?
Deep within the core of personalized recommendations lies the wizardry of advanced machine learning and artificial intelligence (AI). The intricate machinery of this process operates through several key stages:
- Data Collection. The system embarks on data collection, amassing a trove of user information, spanning user interactions, demographics, shopping history, and more. This data forms the bedrock for crafting personalized suggestions.
- Data Preprocessing. Before generating recommendations, the system meticulously preps the data, casting off any extraneous noise or inconsistencies. This ensures recommendations are honed for precision.
- Algorithmic Analysis. Diverse recommendation algorithms come into play, scrutinizing the processed data. These include collaborative filtering, content-based filtering, and hybrid approaches. The analytical engines crunch data to deliver personalized suggestions.
- Personalized Recommendations. Armed with the analysis, the system serves up personalized recommendations, including product recommendations on e-commerce platforms and film or music recommendations on streaming services.
Types of Personalized Recommendations
The world of personalized recommendation engines sprawls across multiple genres, catering to different use cases. Let's traverse the broad categories:
Data-based Product Recommendation Engine — Beyond The Purchase History
The data-based product recommendation engines are pivotal players in personalized suggestions, orchestrating a symphony of user preferences and item characteristics to deliver tailored recommendations. This system manifests the ever-evolving landscape of data-driven insights and machine learning algorithms, redefining how we engage with online stores and content platforms.
For example, the AI Recommender System from Banuba uses contextual bandits and non-negative factorization to process the data gathered and learn whether the suggestion worked based on user interaction.
Content-based Filtering Systems
Content-based filtering systems make recommendations by analyzing the inherent characteristics of items. In the context of e-commerce, if a user frequently buys high-end sports shoes, the system will suggest sports-related products that share similar attributes, such as quality, brand, and price range. These recommendations are rooted in both the user's preferences and the distinctive features of the items themselves.
For content-based filtering systems to function effectively, they rely on a rich dataset encompassing detailed item attributes and comprehensive user profiles. The system then draws connections between user profiles and item attributes to propose products that closely align with the user's preferences. Think of it as having a personal shopper who possesses an intricate understanding of your style and preferences.
Collaborative Filtering Systems
Collaborative filtering systems take a different approach to making recommendations. Instead of focusing on the inherent attributes of items, they rely on users' collective behavior and preferences. These systems are founded on the principle that users who have shown similar preferences or behaviors in the past will continue to do so in the future.
Collaborative filtering techniques, in general, don't require detailed item attributes or characteristics. They primarily depend on user behavior, such as ratings, reviews, or purchase history, to identify patterns and generate recommendations.
In essence, collaborative filtering systems act like a matchmaker, identifying users with similar tastes and suggesting items one user has enjoyed to another with comparable preferences. These systems excel at discovering new items or content that align with a user's interests, often leading to delightful discoveries and enhanced user engagement.
Memory-based Collaborative Filtering
This approach, also known as user-based collaborative filtering, relies on identifying users with similar preferences to the target user. If User A and User B have both liked or interacted with similar items, the system will recommend items that User B has interacted with but User A has not.
For example, if User A and User B have both rated and enjoyed science fiction movies, the system may suggest science fiction movies that User B has highly rated to User A.
Model-based Collaborative Filtering
Model-based collaborative filtering constructs predictive models based on user-item interactions. These models use various machine-learning techniques to understand patterns and relationships within the data. Instead of solely relying on finding similar users, model-based systems can provide more accurate recommendations and handle larger datasets.
For instance, the system may use advanced algorithms to build a model that predicts a user's rating or preference for a particular item based on their historical interactions and the interactions of other users.
Hybrid Recommendation Systems
Hybrid recommendation systems unite multiple recommendation techniques. By marrying the virtues of both content-based and collaborative filtering, these systems endeavor to furnish more precise and varied recommendations, transcending the limitations of individual approaches.
Hybrid recommendation systems provide a well-rounded solution. They can combine the strengths of content-based and collaborative filtering, offering recommendations that cater to different aspects of user preferences. This not only enhances the accuracy of recommendations but also provides a more comprehensive user experience.
Image-based Recommendation Engine
The image-based recommendation engines don't need previous data sets to learn from and evolve. They leverage images to find the perfect fit to match the user's features.
Let's explore how it works on the example of AI Product Recommendation by Banuba. The engine, based on artificial intelligence and machine learning, analyzes the user's individual appearance and offers best-matching items to complement their natural beauty.
The system considers the skin tone, face shape, eyes and hair colors, and other features and offers personalized product recommendations. It's a win-win choice for the beauty and fashion industries. In addition to finding the ideal items, the recommendations encourage customers to try complementary items and, eventually, add them to a shopping cart.
It is built with user privacy in mind. It doesn't collect or store user data, ensuring that customers can shop confidently, knowing their personal information is secure.
This engine is designed to be accessible across various platforms, including mobile devices, desktop computers, and in-browser. This ensures that users can enjoy a seamless and visually enticing shopping experience regardless of their preferred device.
Camera-based Recommendation Engine
In addition to analyzing the uploaded images, the AI Product Recommendation can give product suggestions in a real-life mode via access to the device's camera. In a mere moment after opening the camera and taking a picture, the system will pick up the best-matching items.
Benefits of Personalized Recommendations Any Online Store Can Leverage
Implementing personalized product recommendations can provide a multitude of advantages to online businesses. Here's a deeper look at the key benefits:
Boosts Average Order Value
Personalized recommendations act as the guiding star, leading users towards complementary products or content aligned with their preferences. This often culminates in users making more substantial and valuable purchases, thereby boosting the average order value. With the AI Makeup Recommendation engine from Banuba, brands report seeing a 30% growth in the AOV.
In fact, 54% of retailers surveyed stated that product recommendations were the underlying factors for the average order value growth. According to Salesforce, a click on a personalized product recommendation during a browsing session increases the average order value by 10% compared to non-clicked scenarios.
Improves Conversion Rates
McKinsey claims that using personalized product recommendations and overall personalization can increase the company's revenue by an astonishing 40%. Unsurprisingly, nearly 75% of online retailers stick to personalization on their websites.
Businesses can markedly enhance the likelihood of turning window shoppers into committed customers by customizing recommendations to meet user preferences. These individualized suggestions ease decision-making and eliminate buying hesitations, thus elevating conversion rates.
Tracks Inventory
Personalized recommendation systems act as a beacon, illuminating the path to efficient inventory management. By analyzing user preferences and demand patterns, businesses can optimize their inventory, minimizing waste and ensuring that popular products are readily available.
Automates Manual Work
In the absence of personalized product recommendations, businesses often resort to manual curation and maintenance of product or content suggestions. Personalization systems offer respite, automating these tasks, thereby saving time and effort.
Decreases Cart Abandonment Rates
The abandoned carts lead to a loss of $18 billion for e-commerce annually. A new 2023 research by Baymard Institute states that the average rate reaches 70%.
With personalized product recommendations, you can enable pop-ups with relevant items just as you track the movement to exit the website. This can also improve customer retention, as 56% of consumers are more likely to return to a brand that offers personalization.
Takes User Engagement To The Next Level
Personalized product recommendations maintain users' engagement and interest, making them more inclined to return to the platform. This not only magnifies immediate sales but also kindles customer loyalty. A loyal customer base is more likely to make substantial purchases over time, fortifying customer retention.
For example, with AI Makeup Recommendations, the smart assistant will offer not only relevant products that will match the user's individual features but also suggest trying on additional cosmetic products that can complement the look.
Personalized Recommendations — User Practices
Personalization is not just a theoretical concept; it is reshaping the landscape across various sectors. Let's unravel its impact and triumphs in real-world scenarios:
Video Content and Live Streams
Streaming giants like Netflix harness the power of sophisticated recommendation algorithms to offer a wide range of movies, TV shows, and videos tailored to users' preferences. These systems scrutinize viewing histories, user ratings, and a multitude of factors to offer a seamless and delightful watching experience. The outcome is longer viewing sessions and heightened user satisfaction.
Like Netflix, TikTok's recommendation algorithm considers a user's past interactions with the app. It considers the content users have liked, shared, or interacted with, and the algorithm uses this data to understand their preferences.
Ads and Promos
Online advertising platforms capitalize on personalization to present ads that resonate with users. By considering factors like browsing history, demographics, and interests, these platforms amplify ad engagement and, in turn, the likelihood of conversion. This practice serves the dual purpose of enriching users' advertising experience and enhancing ad campaigns' efficiency.
Google, the search and advertising giant, has mastered the art of personalized ads. By using data on user search behavior, location, and demographics, they serve ads that are not just relevant but also enticing. This personalization significantly boosts ad performance.
Content and News
News websites and content platforms such as Medium are embracing personalization to suggest articles, blog posts, or news stories that align with users' reading histories and interests. This keeps users deeply engaged and encourages them to delve deeper into the content pool. This, in turn, enhances user retention and drives a deeper interaction with the platform.
Retail and Online Shopping
Personalized product recommendations have become the cornerstone of success for numerous brands in the retail and e-commerce sectors. They have revolutionized the way consumers interact with online stores, enhancing user experiences and significantly impacting business outcomes.
In the cosmetics and beauty sector, Sephora is known for its personalized product recommendations. Through an intricate analysis of customers' past purchases and preferences, Sephora suggests beauty products that align with individual tastes. This approach not only boosts sales but also provides customers with a personalized shopping experience. Sephora's success in this realm demonstrates how personalization can make a substantial impact even within a niche market.
Building a site's product recommendation engine from scratch is a time-consuming and pricy delight yet definitely rewarding. Without tech expertise and talent, the development of a tailored solution can take years, taking you down the list of your competitors. A dedicated tech partner with a fully customizable recommendation engine can meet your requirements, deadlines, and budget, turning a customer journey into an unforgettable adventure.