Face liveness detection identifies if the face in front of the camera belongs to the live person helping to prevent face spoofing attacks and ensuring safe biometric authentication and verification.
We’ve partnered with SVORT startup providing our face liveness detection technology for its biometric access management system. Read on to learn how face liveness detection works, the challenges it solves and areas of its application. Watch the video to see how SVORT implements real-time user verification.
How face liveness detection works
Face liveness detection includes computer vision technologies and deep learning algorithms with face detection and tracking at their core. The technology recognizes the presence of a face in real time using a camera and analyzes certain face characteristics to detect liveness.
Active face liveness detection
Live camera face detection identifies the presence of the human face in front of the camera and requests the user to pass the system of dynamic challenges. These challenges are based on user tracking and extracting data about face movements performed in real time, i.e:
Emotional challenges. The system can ask the user to smile, frown, raise eyebrows, tilt, blink and etc.
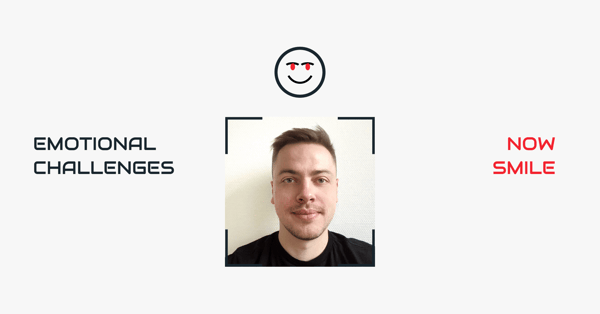
Rotational challenges. The system asks the user to rotate the head in a specific way.

The algorithms analyze if the actions were completed successfully by which the system verifies the individual’s “liveness”. Also, dynamic challenges are chosen randomly making it hard to prepare for spoofing attacks.
Technologies behind liveness detection
-
Emotion expression recognition
The system includes extended set of morphs and anthropometry coefficients by which we can track facial expressions and face triggers of the user (opened mouth, smile, eyebrows raised, winking, etc.).
-
Head pose estimation
The algorithm predicts head tilt and rotation angle and provides the confidence level of the result.
-
Eye-gaze tracking
Banuba has combined machine learning with camera projection methods to create an algorithm capable of tracking gaze vector and point on a handset screen in real time. It detects pupils and irises in two and three dimensions, creating a 3D eyeball model. Our technology can detect whether a user’s eyes are open or closed, and detects line of sight and micro-movements of the eye with subpixel accuracy in real time.
-
Color reflection
The algorithm displays a few random colors on the screen and tracks the color light reflection in the user's eyes.
Additional hidden identification methods
For more user-friendly or continuous authentication, the system may use additional hidden identification methods which involve no action from the user. These methods include:
-
Heart-rate detection (pulsation)
We implement a Colour-Space Super Resolution algorithm that improves colour precision (bits per pixel per channel) at cost of frame rate (by combining aligned consecutive frames). It uses that information to recognize invisible color changes in the human face caused with blood circulation which can’t be reproduced in photos or masks.
-
Single-frame checks
In the case of photo or video, the image grid of the camera overlaps with the printing halftoning dots, and in the case of mobile displays, it overlaps with the pixel grid. Single-frame checks analyze the image to detect these Moire patterns, the angles and other artifacts caused by the overlapping of digital grids.
SVORT: biometric access management system with liveness detection
Our partner SVORT develops a new generation of anonymous biometric authentication system. The system includes a biometrical module which converts the image of the user's face into an anonymous biometric key used to further grant access. In other words, SVORT teaches the system to recognise you each time when you present your face in front of the camera.
Face liveness detection prevents the usage of photo or video for biometric authentication. The input data for face liveness detection block is a video sequence or a series of images. The main tasks are to obtain confirmation that the user is in front of the camera in real-time and to prevent the use of presentation attack instruments (video, photo) to compromise the user's biometric key.
-
“Escape the maze” challenge draws a specific face movement sequence requesting the user to turn the head in a certain way. The algorithms estimate the face angle and head pose at every step making it impossible to spoof the system with a photo or video. This method is unique to the market and by far the most effective for enabling the rotation challenges.
-
The system of dynamic challenges estimates about 12 parametres to detect face liveness with facial expressions like smile, frown, open mouth, line-of-sight, head pose estimation and other face analytics technologies.
-
3 different parameters are generated randomly within a user session. The verification session can be limited within time making it hard for a hacker to prepare presentation attack instruments.
When the user passes “Escape the maze” challenge, the system simultaneously controls additional parameters:
-
heart-rate detection (pulsation)
-
single-frame checks
-
eye pupil position
-
face angle and head rotation
Based on the results of the challenge completion, the system decides whether to continue or terminate further processing of biometric data by other blocks.
Interested to try face liveness detection technology for your business? Contact us!
Why businesses adopt face liveness detection
Businesses and enterprises integrate biometric authentication systems to improve customer service and cybersecurity. Face liveness detection makes biometrics onboarding, account verification and management robust and safe both for businesses and users.
Businesses can register clients or employees by using their facial biometrics and further applying them in a variety of scenarios:
-
Identify employees or clients
-
Grant access to physical premises, objects and analyze their behaviour
-
Distant account registration when the individual submits biometric data to the system via the camera
-
Safe biometric authentication when the user accesses account information
-
Determine the user presence when the individual performs financial transactions
-
Recognize false or duplicate person identities
-
Prevent multiple account registration for one user, fake or duplicate account creations
What are face liveness detection use cases?
The term of liveness detection normally refers to biometric, face recognition and anti-spoofing software. It’s applied in a variety of biometric authentication systems to prevent face spoofing and presentation attacks.
Banking and fintech
Banks and payment systems integrate face liveness detection as a robust way to effectively validate the identities of their clients and ensure safe remove access to their mobile and web services. Clients can use their biometrics (faces) as passwords for more convenient authentication and onboarding.
Liveness detection for face recognition
A wide range of security and law enforcement systems gradually integrate facial recognition technology at large scale. While the accuracy of face identification increases, the majority of facial recognition solutions are still vulnerable to presentation attacks with photos, videos, masks and makeup been used to cheat facial recognition.
Trading platforms and exchanges
Face liveness detection can be integrated into trading and exchange platforms for distant account registration and verification of the account owner, making sure the real person performs finance transactions.
Facial recognition access control systems
Outdoor access control systems can integrate face liveness detection to prevent unauthorized people from entering offices, events, production facilities and locations.
Education
Universities and educational platforms like Coursera can validate the students making sure the real person passes exams or got a certification.
FAQ
What is face liveness detection?
Face liveness detection identifies if the presented biometric (the face) belongs to the authorized, live person captured within the authorization or verification process. It distinguishes the presence of a real person from the presentation or spoofing attack when the user demonstrates a photo, video, or mask to cheat or avoid identification.
What is a face spoof attack?
Face spoof attack or presentation attack is the presentation of an artefact in an attempt to deceive the identification system by presenting a fake biometric parameter (the face).
Face anti-spoofing system is a set of protective measures and techniques used to detect the usage of presentation attack instruments photo, video or mask — and prevent face spoof attacks. Anti-spoofing liveness detection defines whether the face presented in front of the camera is the real person or the presentation attack instrument.
Interested to try face liveness detection technology for your business? Contact us!